Deep Learning Algorithms & 3D Electrodes' Architecture

The knowledge of the complex architecture of Li-ion battery electrodes is crucial both to understand what limits their performance and to simulate their electrochemical properties as accurately as possible. The synchrotron X-ray nano-tomography technique has allowed, with a high spatial resolution (50 nm), the characterization of the 3D structure of Li-ion battery NMC cathodes. The segmentation of tomographic images, a process that separates the different phases present, is a crucial step in image processing that can strongly influence the final result. However, during this step, manual labeling of the images is time consuming and generally difficult to perform because of gray levels alone. It is within the LRCS laboratory in Amiens, that Zeliang et al, have focused their research on the understanding and development of artificial intelligence algorithms to perform this segmentation task automatically, and on several phases simultaneously. This work was done in collaboration with the CMM laboratory of Mines Paris Tech and with Argonne National Laboratory.
The authors proposed in their work published in Nature-NPJ Computational Materials, a Deep Learning approach based on different structures of convolutional neural networks (CNN) of asymmetric depth coder-decoder. A fundamental study about the influence of the most sensitive hyper-parameters was conducted using the SegmentPy software built in A. Demortière’s team and allowing to visualize the activity of neuronal layers. To top it all off, the researchers also showed that the transfer learning approach, that consists of reusing a previously trained network, improves the accuracy of similar data sets. Thus, data from various Li-ion battery materials were successfully segmented with high accuracies (>90%).
Finally, through the supervised approach of CNNs the authors have identified the uncertainties linked to human biases that are diluted in the learning process, and showed the impact of these biases on the uncertainties associated with the 3D structures. In summary, this experimental work combining data collection with original methods of processing observables could accelerate the design of electrodes with ideal porosity and tortuosity for better performing Li-ion batteries.
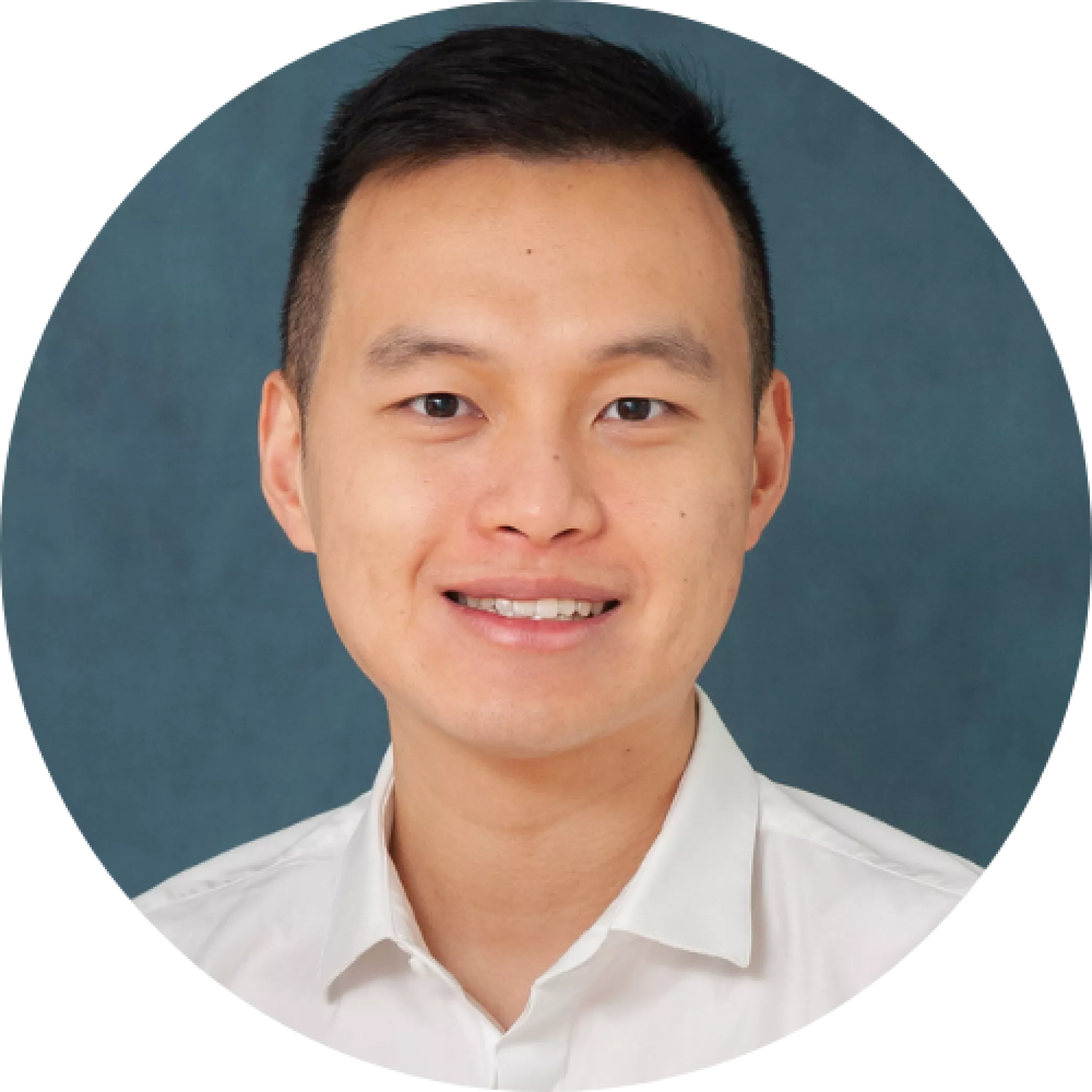
After a bachelor from Measures Physics of I.U.T. in the University of Valenciennes, a Master in Material and Chemistry Engineering from the University Paris Vi, Zeliang Su recently obtained his Ph.D at the LRCS. Before starting his Ph.D., he was involved in two other projects within the RS2E; LTO anode for Li-ion battery with Dr. Damien Dambournet and separator for Li-Sulfur battery with Dr. Mathieu Morcrette and Prof. Claude Guery.
His Ph.D. thesis was under the supervision of Dr. Arnaud Demortière and Prof. Alejandro A. Franco within the LRCS, where they developed ex situ and in situ nano-X ray Computed-Tomography (nano-XCT) experiments using Phase Contrast Techniques to understand the impact of microstructure and transport properties on the lithium batteries. Zeliang has also developed Deep Learning to accelerate the nano-XCT image segmentation.
After the end of his Ph.D, Zeliang is hoping to converge toward the private sector, doing battery research for industry. More specifically, he would like to combine his knowledge of electrochemistry and machine learning by using the latter to accelerate material and battery development. “Working within the RS2E was an excellent experience for me, where I had many opportunities to meet and discuss with brilliant young researchers, and joining on exciting projects.” Respectably, Zeliang has been able to participate in several publications, including four papers as first author.